We’re not interested in tech for the sake of tech. So, there were plenty of skeptics on the team when the possibility of integrating facial identification into our operations came up. Good for iPhones? Yes. Necessary for enrolling recipients in our program? Not obvious. The jury is still out on whether we’ll pursue it at scale, but here is why we decided to run a pilot and what we’ve learned so far:
We recently kicked off operations in a new country and quickly saw some concerning patterns in our data. Prior to signing recipients up for our program, we had sent a team to count households in target villages. When we returned to enroll those households, we saw population numbers 75% in excess of what we’d seen the first time around.
What was driving this sudden spike in residency? Two options seemed likely: (1) community members were showing up in multiple villages to try to qualify for more than one transfer, or (2) legitimate residents were presenting their families as more than one household in order to receive multiple transfers.
As is standard when we see potential fraud, we conducted an independent investigation of suspicious households and in-depth interviews with staff to uncover any malfeasance. But this time around, we added an entirely new approach: facial recognition software. Why did we think this technology might be helpful? To the extent the first form of gaming was at work, running a de-duplication exercise could identify individuals who were trying to “double dip”. We also saw value in the deterrent effect the technology might have on both staff and communities.
So, here’s the process we started testing: We captured photos of recipients during our first touch-point, and sent this data to a technology partner, who ran an algorithmic check, after which a shortlist of ambiguous comparisons were subjected to a human check. If both the automated and human check detected a duplicate, they were sent back to our team for further investigation. We worked closely with the technology partner to ensure that recipient privacy, and biases related to how the AI identifies faces, were mitigated.
What did the results show? To date, detected duplicates have been low. Of >3000 pictures screened, the vendor flagged 150 potential duplicates through the combined algorithm and human screening. Our team further narrowed the pool to 14 likely dupes, and an additional 23 possible (all under investigation). While the numbers weren’t sizable, the cases we did identify provided fairly strong evidence of misconduct on the part of multiple staff members. While this is of course not the news we wanted, it was a valuable outcome, given definitive proof on staff-driven fraud can be hard to come by.
Through on the ground investigation, we’ve since determined the low rate of duplicates largely stems from the fact that most fraudulent activity was of the second variety (i.e. single households pretending to be multiple). We’ve since re-trained our staff to better discern such cases, and are also rolling out stronger messaging in community meetings that high levels of gaming will place the entire village at risk of being disqualified.
So, are we planning to scale the facial ID checks? The technology is clearly not a panacea, but has also shown some demonstrable value. At the moment, we plan to finish out the pilot before we assess the full set of cost benefit trade-offs. We’ll keep you updated.
—
This study is made possible by the generous support of Good Ventures, and the American people through USAID. The contents of this blog are the responsibility of GiveDirectly, and do not necessarily reflect the views of USAID or the United States Government.
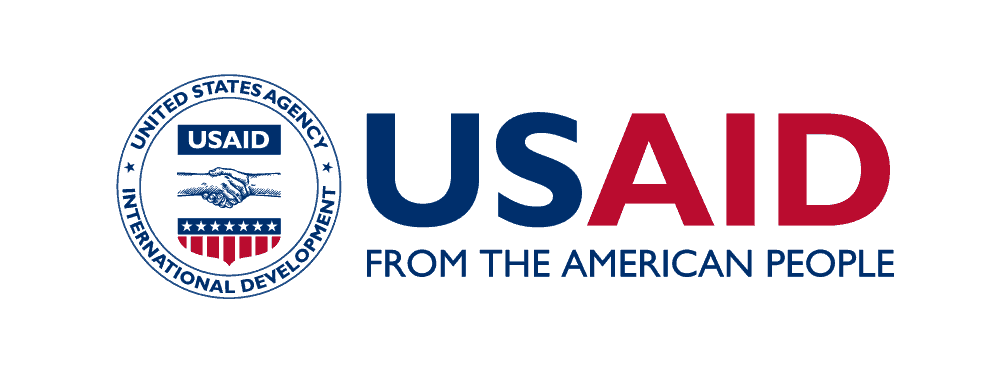